Issue #2: AI snake oil
Like any rapidly growing scientific(-ish) field that captures the public’s imagination, ML is susceptible to its fair share of snake oil salesmen and dubious claims of magical achievements. This issue really crystallized for me with the Siraj Raval scandal last year. Raval is a YouTube personality and self-proclaimed data scientist who makes “Artificial Intelligence education” videos for nearly 700,000 subscribers. His work has been praised and retweeted by Elon Musk and DeepMind’s Demis Hassabis, and his list of followers on Twitter used to include the likes of Elon Musk, Marc Andreessen, Jeff Dean, Ian Goodfellow and many other credible, well-known personalities not just in ML but in tech broadly. While his videos have always had that unmistakable YouTube clickbait-ness to them, Raval’s videos were entertaining and relatively informative for beginners (except for predicting stock prices with 10 lines of Keras code, please don’t do that). All was going well until September 2019, when a reddit post on r/MachineLearning accused Raval of scamming students through his paid course called “Make Money with Machine Learning,” which charged $199 for access. Many students, some of whom were experienced data scientists, found the course to be poorly made, full of mistakes and abandoned by Raval. When students began requesting refunds, Raval quietly edited the course’s refund policy to be “within 14 days of registration” (it was 4 weeks past registration date at that point) [article]. As the ML course fiasco was still in full swing, researcher Andrew M. Webb wrote a Twitter thread showing that Raval almost fully plagiarized a “Neural Qubit” paper and presented it as his own work (the original paper is called “Continuous-variable quantum neural networks”).
Three weeks ago, Raval posted an apology video on YouTube and is now back to making “educational” content. While I believe that people deserve a second chance, there are allegations that Raval still has not fully refunded some students.
What I find interesting about the whole Siraj Raval ordeal is not so much that there are AI snake oil salesmen. Scamming people with a shoddy ML course and plagiarizing papers is appalling, but I don’t think that happens often, especially with such prominent personalities. What does happen on a regular basis is excessive hype around AI/ML (this acronym is an example of that), irresponsible and downright unethical use of ML, and the use of the subject’s technical complexity to obfuscate flaws in research papers. I’m going to break down these statements into examples below, but my takeaway from all of this is that we need to take on more responsibility in countering misleading claims that capitalize on ML’s popularity and complexity. As a first practical step, I am going to follow Vicki Boykis’ 4 excellent suggestions that she wrote about in her newsletter Normcore Tech (one of my favorite newsletters on the tech industry – highly recommend):
We need to provide people with the right tools and content to evaluate what they’re watching education-wise for tech topics
We need to end the hype cycle around AI and ML pronto. I’ve been pretty vocal about this myself, and one of the reasons I started this newsletter - to moderate the gushing fountain of both tech enthusiasm and tech negativity from the mainstream media. [Vicki’s newsletter]
We need to be critical of what we watch and read (easier said than done!)
We need to help people around us if we see they need technical boost, and to make ourselves available. We need to learn to offer constructive criticism outside the parameters of a sarcastic tweet or a one-off YouTube comment. In today’s internet, ultra-engineered for attention and outrage to push all our worst buttons as humans, it can be really hard on a daily basis, but we have to try.
[source]
I’d add one thing to these great suggestions: we need to improve how we promote credible, solid research to make it as appealing to the media and general public as OpenAI press releases. This is hard for many reasons, such as lack of funding, absence of massive PR departments with said funding and, more often than you’d think, reluctance to engage in “marketing” because of a false perception that it is “beneath” serious research (i.e. my work speaks for itself). First, it goes without saying that great marketing is a science and an art. Second, a marketing lens helps to distill the essence of one’s work into familiar and approachable language, far better than even an abstract could do. But, making your voice heard can be difficult, so it’s important to build relationships with the media and get to know the journalists that cover tech. One way to do this is to make yourself available, like Vicki says in #4, to offer constructive criticism and be one of those experts quoted in a NYTimes article about a new ML breakthrough.
I’d love to hear your thoughts on the issues mentioned above and any advice for how to deal with them. If you disagree with anything I wrote, I’d love to have a discussion about that too.
P.S. On Monday, I am starting my last semester of undergrad at Cornell. While I still don’t know what I’ll be doing after graduation, I’m excited for what’s to come, especially in terms of growing and improving Fairly Deep.
Thank you for reading ⚡️
The Horsemen of the Credibility Apocalypse
Right before New Years, a pre-print of a paper made rounds on Twitter because it claimed that YouTube’s new recommendation algorithm has a de-radicalizing influence on users:


Several prominent VCs even proclaimed that the study was an example of a “narrative violation.” It also seemed like one of the authors had an axe to grind with the mainstream media:

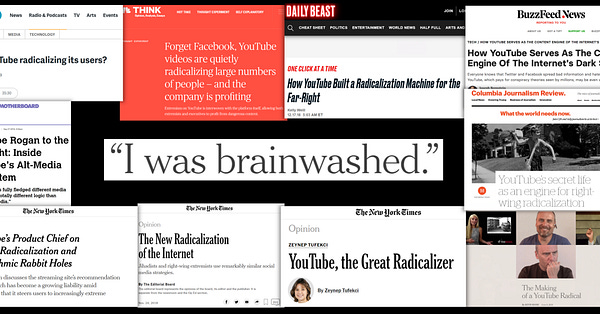
Almost immediately, several acclaimed researchers called into question the methodology of the study. Among them was Princeton’s Arvind Narayanan, a privacy and AI ethics expert, who published a thread examining many of the flaws of this (irreproducible) study, such as this:


Though it’s impossible to tell how many people who saw Ledwich’s thread and paper also saw the criticism, I thought this was a great example of the ML community coming together to refute a clearly flawed paper.
Another interesting case is of the far more credible and serious paper published by Google Health and DeepMind in collaboration with a number of top research hospitals. The paper presents a new ML system that outperforms doctors in detecting breast cancer from mammograms and “reduces false positives by 5.7 percent for US women” and by 1.2 percent in the UK. It also reduced false negatives by 9.4 percent and 2.7 percent in the US and the UK respectively. While these are wonderful results and ML clearly has a lot of potential to improve healthcare outcomes, media coverage of the study was fairly balanced and included cautionary remarks that these AI/ML systems are not be all end all.
From the NYTimes:
in some instances, A.I. missed a cancer that all six radiologists found — and vice versa.
Dr. Lehman [director of breast imaging at the Massachusetts General Hospital], who is also developing A.I. for mammograms, said the Nature report was strong, but she had some concerns about the methods, noting that the patients studied might not be a true reflection of the general population. A higher proportion had cancer, and the racial makeup was not specified. She also said that “reader” analyses involving a small number of radiologists — this study used six — were not always reliable. [article]
In Wired, an award-winning science journalist Christie Aschwanden cautioned that ML systems designed for controversial healthcare practices can exacerbate the problem of “bad medicine”:
In a sense, that’s what happened with the recent Google paper. It’s trying to replicate, and then exceed, human performance on what is at its core a deeply flawed medical intervention. In case you haven’t been following the decades-long controversy over cancer screening, it boils down to this: When you subject symptom-free people to mammograms and the like, you’ll end up finding a lot of things that look like cancer but will never threaten anyone’s life. As the science of cancer biology has advanced and screening has become widespread, researchers have learned that not every tumor is destined to become deadly. In fact, many people harbor indolent forms of cancer that do not actually pose a risk to their health. Unfortunately, standard screening tests have proven most adept at finding precisely the latter—the slower-growing ones that would better be ignored. [article]
👂All Ears: a selection of memorable podcast episodes
Recode’s Kara Swisher needs no introduction. She is one of the most prolific and well-known tech journalists and has been active in the space for over 20 years. She knows everyone in the industry and once made Mark Zuckerberg sweat so much in an interview that he had to take off his hoodie. Her most recent interview with Ben Silbermann, the CEO of Pinterest, offers an excellent look into the company’s efforts to deal with misinformation on the platform and how Pinterest is surviving in a competition with the likes of Facebook, Google, Snapchat and others. [link]

Just For Fun
Bayes 😐 or Bae-yes 🥰?
Before the holidays, the ML community on Twitter and Reddit was consumed by a debate on what should and shouldn’t be considered “Deep Learning.” Now that this is last year’s news, the community is fervently engaged in debating the merits of Bayesian Deep Learning, which is inevitably growing into a frequentist vs. Bayesian reasoning debate. I have no opinion on this because I just heard about Bayesian Deep Learning for the first time when I saw this debate on Twitter, but I do share this sentiment:
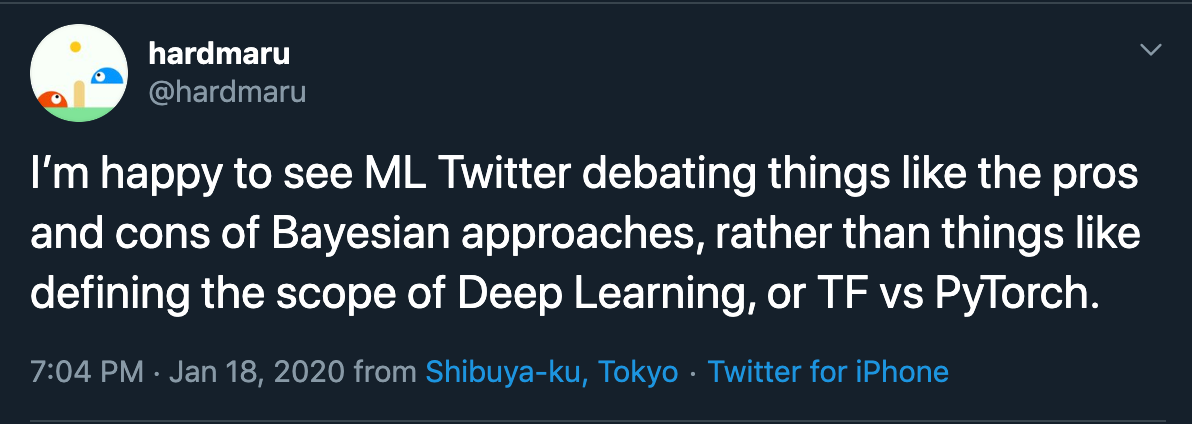
Thank you for reading and see you next time!